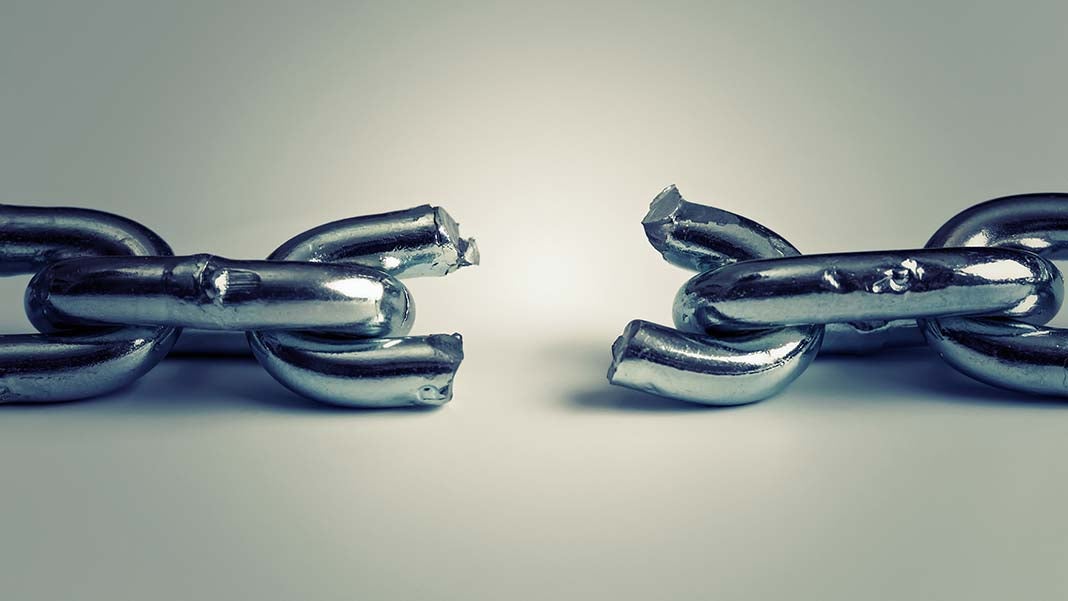
SEOs and marketers, you better hold on to your keyboards, because Google may be about to fundamentally restructure how its search engine goes about indexing web pages. If a team of Google researchers has their way, link profiling may become a thing of the past, replaced by a centralized, Google directed, proto-artificially intelligent algorithm that taps into the company’s vast (and growing) Knowledge Vault to rank websites based primarily on relevance and factual information instead of the number and quality of incoming links.
Isn’t digital marketing fun?
The move would clearly represent a seismic shift in the way information is indexed on the world’s largest search engine which, according to Google’s own numbers, is presently crawling and indexing more than 60 trillion individual web pages to “deliver the best results possible” for its more than 100 billion user searches per month.
Up until now, Google has seemed to operate as if it believes back link data is still the most viable way to ensure quality search results. Links are still a major part of the ranking algorithm, although recent algorithm changes—most notably Google’s 2013 Hummingbird update, which incorporated semantic understanding and contextual relevance (thus the emphasis on quality content) as means of determining search results (and by extension, rankings)—have enabled Google to begin shifting from such external (exogenous) signals like hyperlink structure to internal (endogenous) signals like webpage content.
In simpler terms, Google’s rapidly evolving AI search engine is giving the company the ability to replace its traditional hyperlink structure with factual accuracy as the principal means of determining a website/webpage’s trustworthiness and, by extension, relevance.
I haven’t decided if this is a good or bad thing.
From Linking to Thinking
Even though things are rapidly changing, the Google search engine algorithm of today still relies heavily on the number of incoming links to a web page, coupled with the quality of the links (a link from a higher authority website like the NY Times would be considered of higher quality than a link from a new website) to determine a page’s SERP (search engine results page) ranking.
Over time, this type of link-based classification has birthed a less-than-perfect system that sometimes rewards popular, highly trafficked websites that contain misinformation with higher page rankings than they objectively deserve. In doing so, it’s created a kind of self-perpetuating cycle that constantly feeds on itself, engendering a de facto “if you link to it they will come” mentality. In other words, like many a high school student council election, Google’s search engine rankings often reflect more of a popularity contest than a merit-based system.
Related Article: The Importance of Ranking on Page 1 of the Search Engine Results
To combat this, a Google research team is capitalizing on advances in machine learning to create a new classification system that would measure the objective factual accuracy of a webpage rather than its subjective reputation across the web. Instead of tracking the number of incoming links to a page, the knowledge-based system would count the number of incorrect facts throughout the page and use the results to assign a score, which the Google researchers are calling the Knowledge-Based Trust score.
The Google Knowledge Vault
To function properly, this new system needs free-and-easy access to a large pool of factual information. Enter the Knowledge Vault, a massive, positively Orwellian repository of data Google has been collecting from folks like you and me via its search engine lo these many years. According to Wikipedia, as of 2014, the Knowledge Vault contained 1.6 billion facts which had been collated automatically from all corners of the web.
Whereas its precursor, the Knowledge Graph, was limited to pulling information from trusted crowdsourced sites like Freebase and Wikipedia, Google’s Knowledge Vault is able to tap into the virtually limitless ocean of raw data that is the Internet and then apply advanced machine learning techniques to rank the veracity and relevance of the information.
The concept behind the Knowledge Vault was presented in a recent paper with the attention-grabbing title, Knowledge Vault: A Web-Scale Approach to Probabilistic Knowledge Fusion. It was authored by Xin Luna Dong, Evgeniy Gabrilovich, Geremy Heitz, Wilko Horn, Ni Lao, Kevin Murphy, Thomas Strohmann, Shaohua Sun, and Wei Zhang—all members of the Google research team.
In the first pages of the paper, the authors offer the following as a rationale behind the Knowledge Vault:
“The quality of web sources has been traditionally evaluated using exogenous signals such as the hyperlink structure of the graph. We propose a new approach that relies on endogenous signals, namely, the correctness of factual information provided by the source. A source that has few false facts is considered to be trustworthy…We also observed that Wikipedia growth has essentially plateaued, hence unsolicited contributions from human volunteers may yield a limited amount of knowledge going forward. Therefore, we believe a new approach is necessary to further scale up knowledge base construction. Such an approach should automatically extract facts from the whole Web, to augment the knowledge we collect from human input and structured data sources.”
Welcome to the Knowledge Vault.
Further on in the paper, the Google research team sheds some light on the massive size and scale of Knowledge Vault (KV) as well as the process used for determining the factual accuracy of information:
“KV is much bigger than other comparable KBs (knowledge bases)…In particular, KV has 1.6B triples, of which 324M have a confidence of 0.7 or higher, and 271M have a confidence of 0.9 or higher. This is about 38 times more than the largest previous comparable system…”
{A quick note on “triples.” To determine factual accuracy, Google’s Knowledge Vault looks for information that falls into a pattern of what it calls triples. Triples are made up of three factors: a subject that’s a real-world entity, a predicate that describe some attribute of that entity, and an object that is the value of the attribute. An example of a triple would be that President Obama (subject) is the president (predicate) of the United States (object). Amazingly, the Knowledge Vault contains over a billion triples from across the Internet. As part of the information-culling process, Google’s Knowledge-based Trust algorithm is employed to determine whether particular facts are true and verifiable.}
In fairness, the proposed changes should not come as a surprise to marketers who have been paying attention; the folks at Google have been telegraphing this potential move for months and even years now with numerous search algorithm updates that have progressively emphasized endogenous signals such as quality webpage content over exogenous signals such as link building. They’ve been telling us that as long as we produce quality content that provides relevance and value to our audience, the rest will take care of itself.
In fact, when you stop to think about it, the very phrase “quality content” implies factual content, and thus provides a tidy semantic bridge to the Knowledge-Based Trust algorithm.
Once again, it looks like Google is a few steps ahead of the rest of us…
Redefining Search as We Know It
As inspiring as the prospect of an all-knowing, Google-directed Internet cybercop might be to Google’s hyper-brilliant (and hyper-nerdy) research team, I think the search giant would be wise to tread very lightly on any fundamental reconfiguration of its search algorithm in favor of endogenous (i.e. Google controlled) over exogenous (crowdsourced) signals. This is especially true given the ongoing scrutiny of Google’s monopolistic search practices by European regulators and criticism of the same by industry insiders (evidence of the latter is found in the publication of a recent academic paper indicating that the search giant is allegedly degrading its search results to favor its own properties).
Even though exogenous link building is flawed and may not always reward the most factually accurate sites with higher search rankings, in general, exogenous signals like external hyperlinks utilize a semi-organic, decentralized, and crowdsourced decision-making process to determine a website’s relevance; in so doing, they reflect an imperfect, but ultimately human-driven, collective will.
Fact-based endogenous signals, by contrast, draw from a centralized knowledge base that, though initially derived from humans, is parsed, interpreted, and ultimately directed by a centralized artificial intelligence that is owned by a single entity, in this case Google. It all feels a bit too much like inside baseball for my liking.
At its core, the debate over exogenous vs endogenous search signals raises some philosophical questions that far transcend SEO. It asks us to decide whether we prefer to define credibility by way of cold hard facts or human driven intuition (i.e. trust); whether we should put our faith in a decentralized process or a centralized system; whether we should trust in the objectivity of the machine or the subjectivity of human decision making.
In the end, there may not be much of a debate at all; if Google’s researchers have their way, endogenous search will soon be foisted on us whether we like it or not. When that day comes, marketers would do well to forget link building and fully embrace the Google Knowledge Vault.