Avoiding Unnecessary False Declines: A New Year’s Resolution You Can Keep
By: Debbie Fletcher
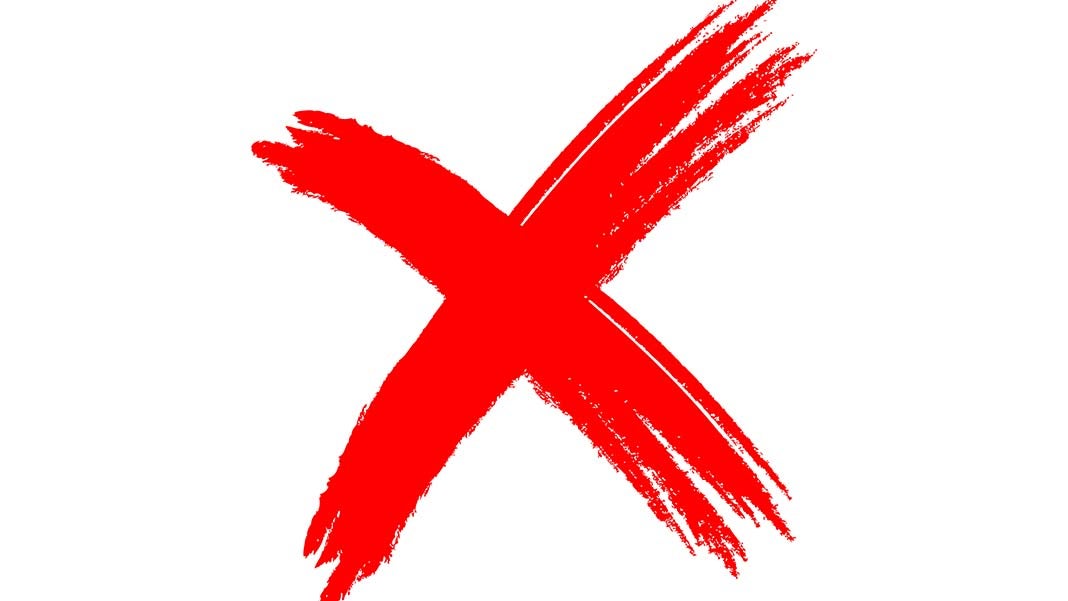
Losing weight is a great New Year’s resolution. Losing revenue? Not so much. The beginning of the new year is a great time to set a new business goal. For eCommerce companies, a good suggestion is reducing the number of orders which are falsely declined by your existing fraud prevention process or solution.
What happens when you reject good orders
Falsely declined orders can bring three levels of pain to your profits: the immediate loss of the rejected shopping cart’s value, the long-term loss of that customer’s lifetime value (shoppers mistaken for fraudsters by a brand rarely return to it), and the wasted cost of acquisition (think of false declines as a leak you’re poking into your own funnel).
But wait, it gets worse. Like an iceberg, the bulk of the problem of false declines is invisible to many online merchants. One reason is the lack of any obvious indication that a certain declined order was in fact legitimate. This is in stark contrast to the notifications, process, and fees associated with chargebacks.
Another reason is the fact that there’s no threshold penalty like there is chargebacks. With chargebacks, many payment processors will stop doing business with a merchant if the chargeback percentage of their total transactions exceeds a defined limit (usually 1%).
As we’ll see below, when there’s no clear penalty for falsely declining an order, inadequate and outdated ecommerce fraud prevention tools end up becoming widely adopted.
Lastly, since an e-tailer’s total rate of declined orders is usually in the low single digits, even if all of those were false declines, the short-term impact of the problem is incremental when compared a brand’s bottom line. Thus, there is little immediate incentive to expend resources towards recouping that lost revenue.
The “invisibility problem” of false declines naturally causes merchants to feel that far more of their declines are fraudulent orders than actually are, due to the fact they are ignorant of the true magnitude of this problem. It’s eCommerce meets the Dunning-Kruger effect.
All this leads merchants to focus on more visible metrics like turnaround time, chargeback rates, and chargeback losses. With so much emphasis put on avoiding chargebacks, overly risk-averse fraud prevention “solutions” become the norm, in-house fraud prevention teams stay understaffed, under-equipped, and overworked. In such an environment, little to no effort is put into looking beneath the surface of a borderline or “risky” order.
Blacklists are Ineffective
One tool in particular contributes to many false declines: e-commerce fraud prevention blacklists. These are essentially lists of names, shipping addresses, and other order details which have been associated with a chargeback. When new orders come in, the order details are checked against this blacklist, and if one or more of the order’s details match the list, that order can be automatically declined.
Although they seem like a quick, efficient, and accurate solution, there are many reasons why blacklists can block good orders and end up doing more harm than good. One of them is the fact that a single shipping address can be legitimately used by many people (think re-shippers and university dorms). If one person at such an address, at one point in the past, initiated a chargeback, all future orders could get needlessly rejected.
Another drawback to blacklists is their tendency to include entire countries. Think about that: an entire nation could be cut off from a merchant because a single order resulted in a chargeback.
Not only are blacklists too blunt of a tool, they’re also ineffective—fraudsters just adapt their tactics or buy new stolen card info on the black market which doesn’t match anything on the blacklist. Blacklists only deliver false declines and a false sense of security.
Why merchants are turning to models
But there’s a better way: adaptive fraud prevention tools based on data modeling. By linking the details on the order to data from social media, public listings and other sources and employing machine learning algorithms, the current best-of-breed solutions on the market today work by reconstructing the likely story behind the order instead of basing the entire accept/decline decision on a few data points. There is a paradigm shift in the industry towards connecting the dots and away from finding a “smoking gun.”
A new year calls for new thinking about combating eCommerce fraud. Make it your resolution to stop leaving money on the table.
3828 Views