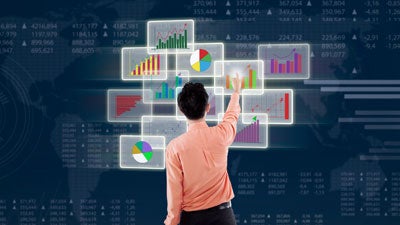
E-commerce, banking, tourism, gaming, manufacturing…These days, data science seems to be everywhere. And, as is often the case with hugely popular technological trends, it is very divisive. Some claim that data science is a fail-proof answer to every business challenge. Others underestimate its significance, believing that it’s nothing short of a fad.
Both sides are wrong. Data science certainly isn’t the ultimate solution, seeing that only 13% of data science projects go beyond the concept stage. At the same time, with a trillion megabytes of data produced every day, the technology potential is tremendous. The demand for trained specialists is very much present and sure to grow.
The following data science myths aren’t the only ones technology experts need to confront day after day. Unfortunately, similar fallacies deter industry leaders from investing in data research and may dishearten potential data scientists from pursuing a career in this field. In this article, experts from Itransition discuss — and debunk — the six most notorious misconceptions about the profession of a data scientist.
1. Data science is just a fad
We already mentioned this one in the introduction, but it’s important to set it straight: data science is and will continue to be relevant in the foreseeable future.
To begin with, it’s nothing new. Data science has been around at least since the 1960s, practiced by statisticians and economists in one form or another. However, two things have changed since, leading to the development of a new field: the wave of digitalization and the resulting rise in the volume of data.
These two processes are still underway, at an even faster pace than before. The increasing presence of the Internet of Things and Big Data, in particular, is why the need for data science will prevail and expand.
2. Data scientists are software engineers
Since both professions operate on the intersection of programming, analytics, and even economics, it’s easy to see why they are often confused. Despite some resemblance, however, the two occupations are quite different.
Coding is the cornerstone of software engineering. Software developers apply the principles of programming to design, develop, and test software. To do that, they rely on the analyses of user requirements and estimates of business outcomes.
On the other hand, data scientists are tasked with finding ways to translate data into tangible results. They collaborate with various teams within the organization to better understand its goals and framework. Data scientists build models and algorithms rather than software, and, unlike software engineers, they aren’t primarily concerned with writing code.
This brings us to the next myth, and that is…
3. Tools and coding are everything in data science
Sure, fluency in programming languages such as Python, R, or SQL won’t hurt — actually, it helps a lot and is required for most data science job openings. That said, coding alone won’t get you hired if you are looking to grow in the data science field. The same goes for proficiency in popular software like SAS or Tableau.
It’s important to understand that even if an aspiring data scientist knows the required tools inside out, that knowledge needs to be complemented by sound analytical and mathematical background, business acumen, and problem-solving abilities. Soft skills are also vital to be able to share insights with others within the company.
While programming remains a vital competence in data science, this may change in the years to come. That’s because more and more algorithms are coded and made available online, and the capabilities of automated solutions like Google Cloud ML or DataRobot are constantly increasing.
4. AI will replace data scientists
Data science is one of the fields that can greatly benefit from automation to handle mundane, repetitive tasks prone to human error. However, what data scientists lack in pure processing power they make up for in creativity, complex thinking, and knowledge.
Algorithms are unable to make business-driven decisions or account for intricate context. Also, human judgment is necessary to balance out the organization’s goals with the insight of data research and make ethical decisions.
Lastly, a human is simply needed to oversee, control, and keep the machines running. Due to all that, data scientists won’t be replaced by AI — at least not anytime soon.
5. Only large organizations work with data science
Another common misconception is that data science is reserved for big organizations that can afford to spend a fortune on resources. Believing so may discourage future data scientists who prefer to work in a more agile, non-corporate environment.
This myth partially stems from the misbelief that more data equals higher data model accuracy and that it’s only the giants that can afford to acquire, store, and process huge data volumes. In reality, it’s more about the quality of data and how it corresponds to the organization’s needs.
If applied correctly, better technology will usually yield better results. But that’s not to say that smaller businesses that don’t have access to it are excluded from leveraging data. On the contrary, small-scale projects using open-source tools can be both possible and profitable. Limited resources can spark the creativity of many data scientists, leading to a more rewarding and satisfying work experience.
6. A scientific degree is a must
Those who dream of launching a career in data science may be reluctant to do so for one more reason: the lack of a degree in a respective field.
They’ll be relieved to hear that an estimated 25% of data scientists don’t have a Master’s or PhD degree. That’s possible because data science is an exceptionally multidisciplinary field. Business savvy, communication skills, knowledge of tools and programming, and curiosity can all be just as important as formal education. Many pursue data science certification to satisfy their education goals instead of a post-graduate degree.
While the academic background is hugely beneficial, it can’t be removed from reality. Understanding statistics and math is essential but should be treated just like a tool rather than an end in itself. Likewise, theoretical knowledge is useful as long as it aids in achieving business goals rather than following hypothetical scenarios.
Conclusion
Making it in data science is no easy feat — even more so with this many misconceptions around it. Despite that, the field continues to develop. Hope the dispelling of the myths will be a productive first step on your way to becoming (or finding) a data scientist.
2067 Views